Institute for Nonlinear Science University of California San Diego La Jolla CA 92093-0402 Dated. This article is set up as a tutorial for nonlinear principal components analysis NLPCA systematically guiding the reader through the process of analyzing actual.
Pdf Principal Components Analysis With Nonlinear Optimal Scaling Transformations For Ordinal And Nominal Data Semantic Scholar
The authors provide a didactic treatment of nonlinear categorical principal components analysis PCA.
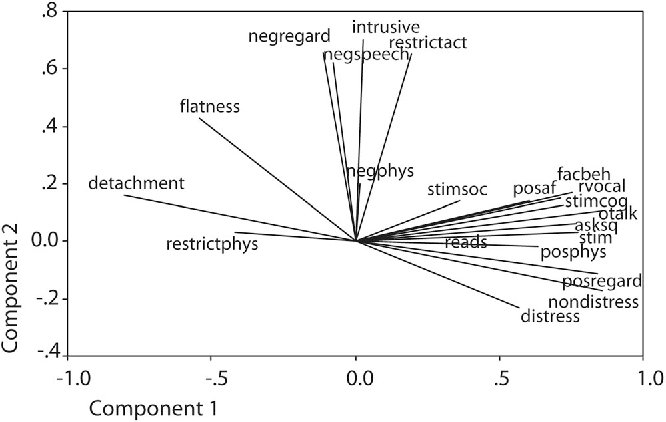
. Principal Component Analysis PCA is a linear dimensionality reduction technique that can be utilized for extracting information from a high-dimensional space by projecting it into a lower-dimensional sub-space. In this chapter we give a brief outline of the methods of data analysis used from elementary T-scores to nonlinear principal component analysis PCA including data normalisation quantification of categorical attributes categorical principal component analysis CatPCA sparse PCA the method of principal variables the original double Kaiser selection. The goal of principal components analysis is to reduce an original set of variables into a smaller set of uncorrelated components that represent most of the information found in the original variables.
Nonlinear principal components analysis with CATPCA. A tutorial Journal of Personality Assessment. The most important advantage of non-linear CATPCA over the linear PCA method is that it combines nominal and ordinal variables and can discover nonlinear relationships between variables.
The non-linear CATPCA method is the nonlinear equivalent of the PCA method to reduce dimensions in categorical data. A tutorial This article is set up as a tutorial for nonlinear principal components analysis NLPCA systematically guiding the. I would suggest having a look at Linting Kooij 2012 Non linear principal component analysis with CATPCA.
NLPCA is a more flexible alternative to linear PCA that can handle the analysis of possibly nonlinearly related variables with different types of measurement level. This method is the nonlinear equivalent of standard PCA and reduces the observed variables to a number of uncorrelated principal components. Abstract This article is set up as a tutorial for nonlinear principal components analysis NLPCA systematically guiding the reader through the process of analyzing actual data on personality assessment by the.
Categorical principal components analysis is also known by the acronym CATPCA for categorical principal components analysis. A Tutorial Journal of Personality Assessment 94 1. Starting from a set of items drawn from a questionnaire we wanted to see whether the construct of job satisfaction consists of one or more sets of components and.
THE METHOD OF NONLINEAR. Ordinal and the researcher is concerned with identifying the underlying components of a set of variables or items while. NLPCA is a more flexible alternative to linear PCA that can handle the ana.
This method is the nonlinear equivalent of standard PCA and reduces the observed variables to a number of uncorrelated principal components. Reducing the number of variables of a data set naturally comes at the expense of. You can create one manually by copying the eigenvalues out of the Model Summary table in the output or if you will need to create a lot of scree plots you can use the SPSS Output Management System OMS to automate pulling the values out of the table and creating the plot.
Principal Component Analysis or PCA is a dimensionality-reduction method that is often used to reduce the dimensionality of large data sets by transforming a large set of variables into a smaller one that still contains most of the information in the large set. The subscales of job satisfaction are computed as the simple sums of the transformed valuesvariablesrotatedspecificcomponent. Psychological Methods v12 n3 p336-358 Sep 2007.
Return to the SPSS Short Course. This article is set up as a tutorial for nonlinear principal components analysis NLPCA systematically guiding the reader through the process of analyzing actual data on personality. This chapter focuses on the analysis of ordinal and nominal multivariate data using a special variety of principal components analysis that includes nonlinear optimal scaling transformation of the variables.
The goal of this paper is to dispel the magic behind this black box. The most important advantages of nonlinear over linear PCA are that it incorporates nominal and ordinal. The authors provide a didactic treatment of nonlinear categorical principal components analysis PCA.
In this type of plot a v ariable is represented by a vector an arrow. Categorical Principal Components Analysis CATPCA with Optimal Scaling. The principal component space where the axes are given by the principal com-.
The method is particularly suited to analyze nominal qualitative and ordinal eg Likert-type data possibly combined with numeric data. The most important advantages of nonlinear over linear. This method is the nonlinear equivalent of standard PCA and reduces the observed variables to a number of uncorrelated principal components.
Request PDF Nonlinear principal components analysis with CATPCA. Nonlinear Principal Components Analysis with CATPCA. Version 2 Principal component analysis PCA is a mainstay of modern data analysis - a black box that is widely used but poorly understood.
This article is set up as a tutorial for nonlinear principal components analysis NLPCA systematically guiding the reader through the process of analyzing actual data on personality assessment by the Rorschach Inkblot Test. Since the early 1930s classical statistical methods have been adapted in various ways to suit the particular characteristics of social and behavioral science. CATPCA does not produce a scree plot.
Categorical principal components analysis CATPCA is appropriate for data reduction when variables are categorical eg. Discussion The aim of this study was to identify the component structure of job satisfaction in the Italian social service sector by analyzing survey-data using nonlinear principal components analysis nonlinear PCA. This method is the nonlinear equivalent of standard PCA and reduces the observed variables to a number of uncorrelated principal components.
It tries to preserve the essential parts that have more variation of the data and remove the non-essential parts with fewer variation. Nonlinear principal components analysis with CATPCA. Published 1 September 2007.
The most important advantages of nonlinear over linear PCA are that it. The authors provide a didactic treatment of nonlinear categorical principal components analysis PCA. The authors provide a didactic treatment of nonlinear categorical principal components analysis PCA.
Relevant clinical information can influence the diagnostic probability and reporting of ultrasound findings. Nonlinear principal component analysis also known as CATPCA can be used instead of traditional PCA to explore nonlinear relationships in cases for which the data are comprised of both qualitative ie nominal and ordinal variables and quantitative variables Linting and van der Kooij 2012 For probabilistic monitoring of nonlinear processes the traditional probabilistic. 101080002238912011627965 PubMed CrossRef Google Scholar Martinez Diego Borja Tathiana Nadin MedellÃn and Pedro Cueva.
Pdf Nonlinear Principal Components Analysis Introduction And Application Semantic Scholar
Pdf Nonlinear Principal Components Analysis Introduction And Application Semantic Scholar
Pdf Nonlinear Principal Components Analysis Introduction And Application Semantic Scholar
Pdf Nonlinear Principal Components Analysis Introduction And Application Semantic Scholar
Pdf Nonlinear Principal Components Analysis Introduction And Application Semantic Scholar
Pdf Nonlinear Principal Components Analysis Introduction And Application Semantic Scholar
Pdf Nonlinear Principal Components Analysis Introduction And Application Semantic Scholar
0 comments
Post a Comment